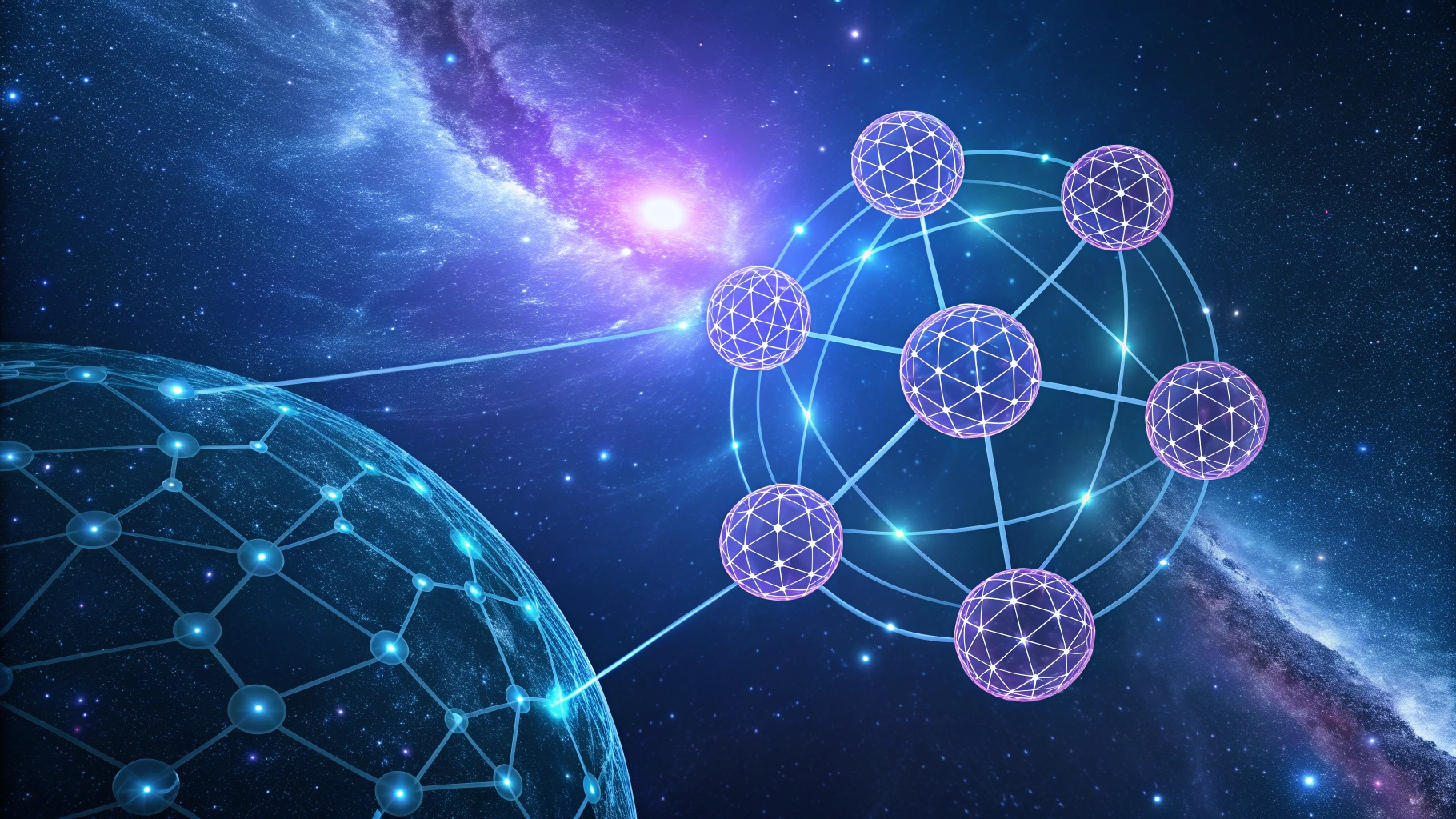
"The most profound technologies are those that disappear. They weave themselves into the fabric of everyday life until they are indistinguishable from it." — Mark Weiser
In a quiet research lab at a prestigious university, a group of scientists recently discovered something unexpected: our fundamental classification systems—the mental frameworks we use to understand the world—might be more fluid than we believed. Additionally, artificial intelligence is accelerating this realization at an unprecedented pace.
Just as astronomers have debated whether Pluto is a planet or whether moons should be classified as planets themselves, we now face similar taxonomical questions about the intelligent systems we're creating. Are they tools or collaborators? Extensions of human intelligence or something entirely different? The answers aren't merely academic—they influence how we integrate these technologies into society and how we prepare for their evolution.
These questions reflect a deeper pattern in human understanding: our tendency to create rigid categories that eventually prove inadequate as our knowledge expands. And AI is forcing us to rethink these boundaries more rapidly than any technology that has come before.
The Categorical Imperative: Why Our Minds Love Boxes
Humans are natural taxonomists. We instinctively categorize everything we encounter—it's how we make sense of a complex world. This cognitive tendency helped our ancestors distinguish between poisonous and edible plants, friend and foe, as well as safe and dangerous environments.
But our classification systems come with two significant limitations:
- Cultural and historical bias: Our categories reflect the time, place, and culture in which they were created
- Resistance to change: Once established, taxonomies become embedded in our institutions and thinking, making them challenging to revise even when new evidence demands it
Consider how we've historically classified planets. For centuries, astronomers defined planets based on what was visible to the naked eye. Then, telescopes revealed new celestial bodies, leading to a taxonomical crisis. Similarly, AI is pushing us beyond the comfortable categories we've used for a long time to understand technology. One AI researcher noted, "It's like we've been arguing about whether Pluto is a planet while a completely new kind of astronomical object has appeared in our telescopes."
The False Dichotomy: Tools vs. Intelligence
When we think about artificial intelligence, we often fall into a binary trap: AI systems are either just sophisticated tools or they are approaching human-like intelligence. This dichotomy reflects historical debates about celestial bodies: something is either a planet or it isn't. But what if this framework itself is inadequate?
Consider the following problem:
"How should we classify a machine learning system that can diagnose diseases more accurately than human doctors in some contexts, but completely fails to recognize basic patterns that any medical student would catch in others?"
This doesn't fit neatly into our tool/intelligence dichotomy. Instead, we might need new taxonomical approaches:
- Recognize that AI systems exist on a spectrum of capabilities rather than in discrete categories
- Acknowledge that intelligence takes many forms, both human and non-human
- Develop classification systems grounded in capabilities and contexts instead of anthropocentric comparisons
- Accept that our taxonomies will continue to evolve as AI systems advance.
Just as planetary scientists now consider factors like geological complexity rather than just orbital dynamics, we need frameworks for AI that capture their multidimensional nature.
The Two-Phase Approach: How We Might Reclassify AI
To address these taxonomical challenges, we might adopt a two-phase process similar to how scientists have approached planetary classification:
Phase 1: Decomposition
Rather than viewing AI systems as monolithic entities, we can break down their capabilities and impacts into more specific components:
- Domain-specific capabilities: What tasks can the system perform within defined boundaries?
- Cross-domain adaptability: How effectively does the system transfer learning across different contexts?
- Human-AI interaction patterns: How do humans collaborate with or rely on the system?
- Societal implications: What broader impacts does the system have on communities and institutions?
This decomposition helps us move beyond simplistic questions like "Is this AI intelligent?" to more nuanced assessments of specific capabilities and impacts.
Phase 2: Integration
The second phase involves creating new frameworks that integrate these decomposed elements:
- Developing multi-axis classification systems that capture the complexity of AI capabilities
- Creating contextual frameworks that recognize that an AI's category might shift depending on its application
- Building dynamic taxonomies that can evolve as AI technologies continue to develop
Traditional AI Classification:
Narrow AI → General AI → Superintelligence
Focused primarily on comparison to human intelligence
Multi-dimensional Approach:
Capability Profiles × Domain Expertise × Interaction Models × Impact Assessments
Recognizes the complex, multifaceted nature of AI systems
This integrated approach allows us to assess AI systems more accurately and prepare more effectively for their continued evolution.
Beyond Presentism: Learning from Historical Patterns
One of the most instructive aspects of astronomical taxonomy is how often scientists have fallen into the trap of "presentism"—interpreting historical developments through the lens of current understanding. We are at risk of making the same error with AI. Current debates about whether large language models "really understand" language or are "merely statistical systems" mirror historical discussions about whether Pluto is "really a planet." In both cases, we might be asking the wrong questions. A more productive approach could involve:
- Historical perspective: Recognizing that our current taxonomies are temporary stages in an ongoing evolution of understanding
- Pragmatic classification: Focusing on frameworks that serve scientific and social purposes rather than seeking "true" categories
- Taxonomical pluralism: Accepting that different classification systems might be valid for different purposes
- Intellectual humility: Acknowledging the limitations of our current frameworks and remaining open to radical revisions
As one AI ethicist observed: "The question isn't whether our AI classifications are 'correct'—it's whether they help us anticipate challenges, distribute responsibility appropriately, and maximize beneficial outcomes."
The Governance Implications: Categories Matter
How we classify AI systems has profound implications for how we govern them. Consider these divergent approaches:
Tool-Based Governance
If we classify AI systems primarily as tools, governance focuses on:
- Product safety standards
- Technical reliability measures
- Clear lines of human responsibility
Agent-Based Governance
If we classify more advanced AI systems as autonomous agents, governance might include:
- Behavioral boundaries and constraints
- Rights and responsibilities frameworks
- New legal and ethical paradigms for non-human entities
The taxonomies we choose don't just reflect reality—they help create it by shaping regulatory approaches, investment priorities, and public expectations.
The Path Forward: Adaptive Taxonomy
Just as planetary science has evolved toward more nuanced classification systems that acknowledge the diversity of celestial bodies, we need AI taxonomies that:
- Embrace complexity: Go beyond binary thinking to acknowledge the multidimensional nature of AI systems.
- Remain adaptable: Create frameworks that can evolve as technologies advance and our understanding deepens.
- Serve human needs: Concentrate on classifications that enable us to use AI systems responsibly and beneficially.
- Maintain perspective: Remember that categories are tools for understanding, not fixed aspects of reality.
The most valuable approach may be what we could refer to as "adaptive taxonomy"—classification systems that explicitly recognize their own provisional nature and incorporate mechanisms for evolution.
Conclusion: Beyond Categories
The debate over whether moons should be classified as planets reveals something profound about human understanding: our categories are not discoveries about the world but creations that help us navigate it. They succeed not when they capture some absolute truth but when they promote deeper understanding and more effective action.
As AI continues to evolve in unexpected ways, our greatest challenge may not be fitting these systems into our existing taxonomies but rather developing the cognitive flexibility to create new frameworks when necessary. The question isn't whether our categories are correct—it's whether they are useful. Perhaps the most important lesson from both planetary science and AI development is the value of taxonomical humility—recognizing that our classifications are always works in progress, reflecting our current understanding while remaining open to revision as our knowledge expands.
Ultimately, the most valuable contribution AI might make to human thought isn't just the problems it solves or the tasks it automates—it's the way it compels us to reconsider our fundamental categories and develop more flexible, nuanced ways of understanding our increasingly complex world.
Inspired by: "Moons Are Planets: Scientific Usefulness Versus Cultural Teleology in the Taxonomy of Planetary Science" by Philip T. Metzger et al.